A/B testing is a widely used method in data-driven decision-making, particularly in digital and in-store marketing, product development, and user experience optimization. It involves comparing two versions of a webpage, app, or other digital content (A and B) to determine which version performs better in terms of predefined metrics. Retail regression analysis, on the other hand, is a statistical technique used to identify relationships between variables and make rollout predictions. Taken together, these two models create a robust test and learn platform for retail testing.
Retail A/B testing in simple terms
Retail A/B testing involves randomly splitting a sample of users into two groups: one exposed to treatment A and the other a control treatment B. By comparing the outcomes of these two groups, the experimenters can identify which treatment leads to better outcomes. A/B testing is particularly effective when assessing discrete changes, such as the impact of an online advertisement on in-store foot traffic.
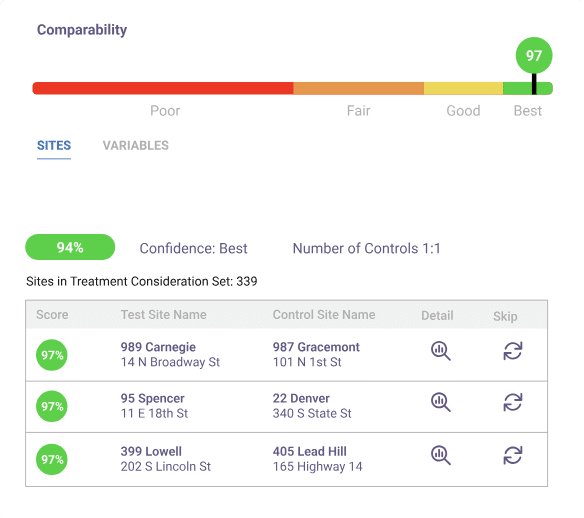
Retail regression analysis in simple terms
Regression analysis encompasses a range of techniques aimed at identifying relationships between variables and predicting outcomes. It can handle more complex scenarios where multiple variables influence an outcome simultaneously. By quantifying the relationships between independent variables and the dependent variable, regression analysis provides a better understanding of the factors — such as demographic buying behaviors — driving observed changes.
Why both matter to retail data and decisioning
1. Multivariate Analysis: A/B testing often focuses on a single treatment variation, neglecting potential interactions between multiple variables. Regression analysis can handle multiple independent variables simultaneously, enabling the identification of combined effects that A/B testing might overlook.
2. Continuous Variables: A/B testing typically deals with binary or categorical changes. Regression analysis accommodates continuous variables, allowing for more nuanced insights, such as the impact of price changes on sales volume.
3. Control for Confounders: Regression analysis enables the control of confounding variables, reducing bias and enhancing the accuracy of conclusions drawn from A/B tests.
4. Assumptions: Retail regression analysis assumes relationships are linear and may not capture non-linear effects. A/B testing, on the other hand, is not as constrained by this assumption.
How applying both models works with test and learn
Imagine a retailer decides to test geofencing to see if the targeted advertisements lead to conversions. They first opt to implement A/B testing to measure the impact on conversions, and they are surprised to find there is a slight reduction in lift. Then they run a regression analysis and discover there is an increase in conversions in high-income areas but a significant decrease in conversions in low-income areas. Consequently, they decide to implement geofencing only in high-income locations.
At MarketDial, we have tools to guide retailers through this process from beginning to end. With the MarketDial app, retailers can set up test groups, control groups, confidence levels, and test lengths for A/B testing. Following the test, they can conduct various retail regression analytics:
Driver Analyzer
A driver analyzer explores what attributes are driving the test outcomes.
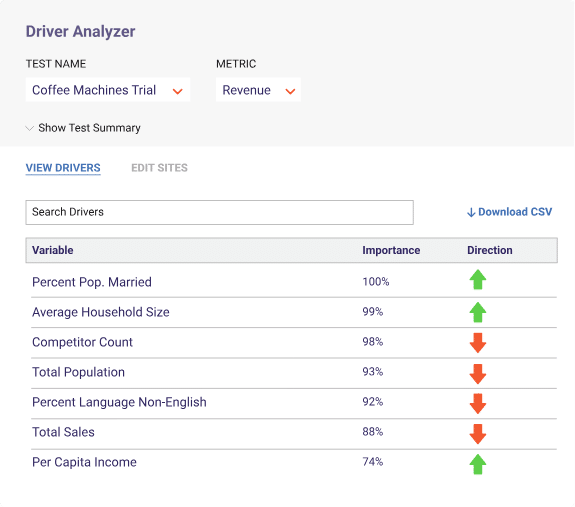
Basket Analyzer
Basket Analyzer details how test variables influence co-purchased items.
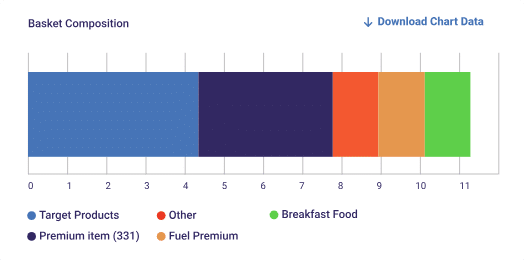
Impact Analyzer
An impact analyzer helps determine what the optimal rollout will be.
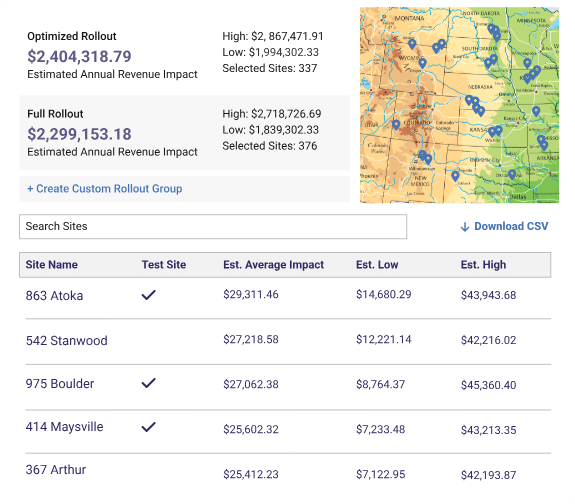
Interested to learn more about regression analysis and how MarketDial’s testing platform can support you? Check out these resources:
The 3 key questions to ask after every in-store test
The rhyme and rhythm of retail test implementation
What is test and learn?